esci:
Estimation Statistics with Confidence Intervals


esci provides student-friendly tools for estimation statistics:
- effect sizes with confidence intervals for many research
designs
- meta-analysis
- visualizations emphasizing effect sizes and uncertainty
- strong hypothesis testing with interval nulls
esci is both an R package and a module in jamovi. If you’re looking for the R
package, stay here. If you want esci in jamovi, download and install
jamovi and then
use the module library to add esci.
Leave comments, bug reports, suggestions, and questions about esci here
esci is still under development; expect breaking changes in
the future especially for the visualization functions. If you
need production-ready estimation, turn to statpsych
esci is built on top of statpsych and metafor.
That is, almost all of the statistical calculations are passed off to
these packages. The only exception is for confidence intervals for
Cohen’s d (see documentation). Why does esci exist, then?
- To provide a design-based approach; each function in esci is for one
type of research design (e.g. two groups with a continuous variable); it
provides all the effect sizes relevant to that design in one convenient
function (e.g. mean difference, cohen’s d, median difference,
ratio of means, ratio of medians).
- To make visualization easier; esci provides visualizations that
emphasize effect sizes and uncertainty
- To integrate with GUIs for students; esci integrates into jamovi and (very soon) JASP.
- To support student learning, from intro to advanced levels; esci is
extensively used in the recently released second edition of our intro
textbook (https://thenewstatistics.com/itns/) and should be ideal
for use in higher level and graduate courses.
The visualizations produced by esci are exquisite in a large part
because of the lovely ggdist package by
Matthew Kay.
Installation
esci is availableon CRAN; you can install with:
Or, get the stable branch directly from github
# install.packages("devtools")
devtools::install_github('rcalinjageman/esci')
Or, try out the development branch:
# install.packages("devtools")
devtools::install_github('rcalinjageman/esci', branch = "development")
Roadmap
- Finish writing documentation and tests
- Review all functions for consistency of parameter names and returned
object names
- Rewrite visualization functions completely to remove clunky
approaches to the difference axis and other issues
- Complete JASP integration
- Rewrite jamovi integration
- Add prediction intervals for basic designs
- Repeated measures with 1 IV and multiple groups
- Fully within-subjects 2x2 design
- Arbitrarily complex designs
Example
library(esci)
data("data_kardas_expt_4")
estimate <- estimate_mdiff_two(data_kardas_expt_4, Prediction, Exposure)
plot_mdiff(estimate)
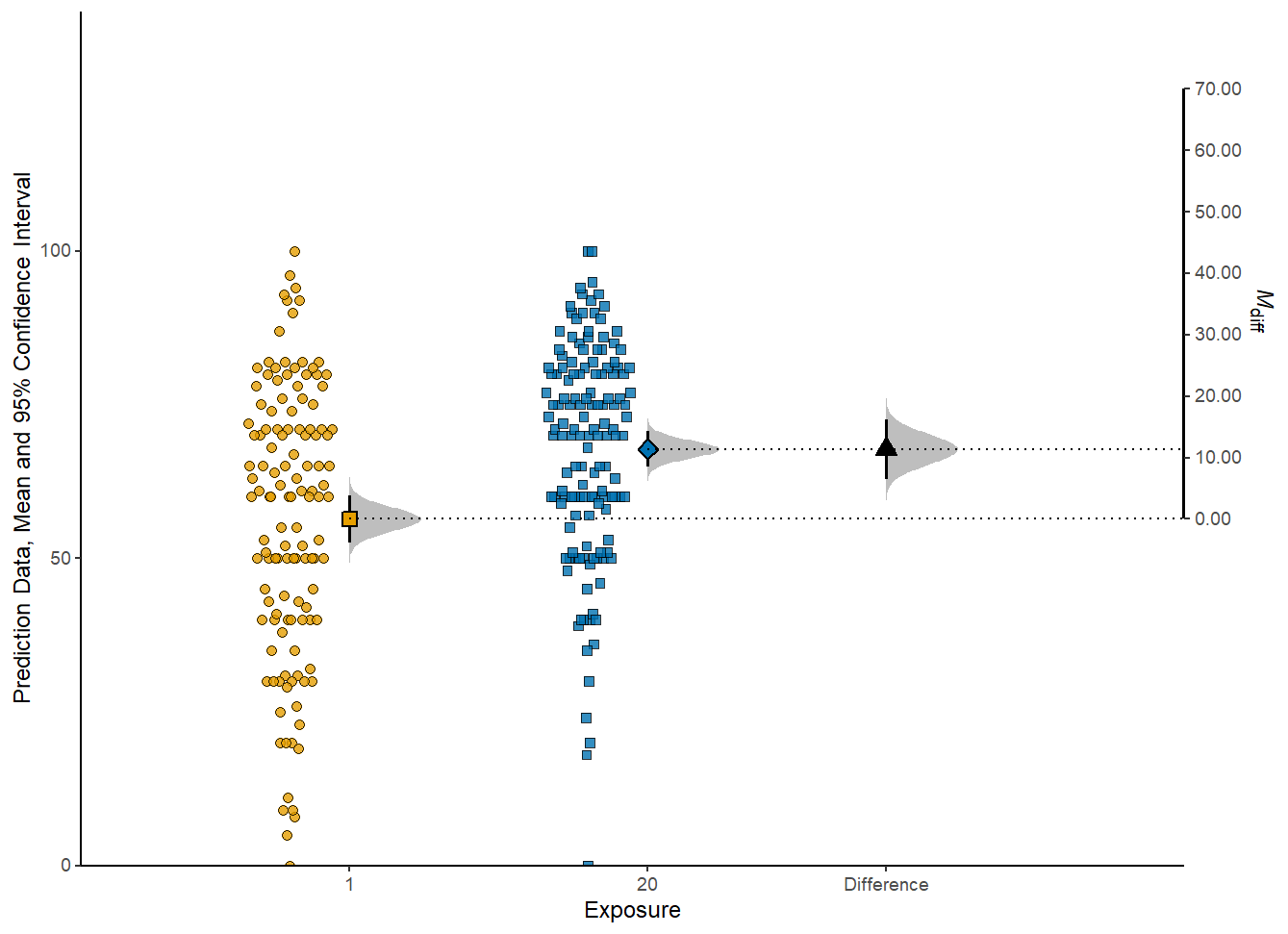